The session was attended by over 1,550 delegates from 50 countries participating in the Africa Law Tech Festival 2024. The speaker was Ommo Clark, Centre for Applied AI.
Watch the Full Video HERE
What exactly are deepfake technologies? A deepfake is a video, image, or audio recording that appears authentic but has been artificially manipulated using AI. These technologies gained widespread recognition in the mid-2010s, with a major breakthrough being the development of neural networks and the introduction of Generative Adversarial Networks (GANs) in 2014. Pioneered by Ian Goodfellow and others, GANs laid the foundation for deepfake technology, enabling increasingly sophisticated and realistic manipulations. Much like Photoshopping in the 21st century, deepfakes rely on deep learning, a subset of AI, to create convincing images of fabricated events—hence the name "deepfake."
Initially, creating deepfakes required significant computational resources and technical skill, limiting their production to researchers and enthusiasts. Early examples were interesting but often lacked the realism we see in today’s deepfakes. Thanks to advancements in AI algorithms, greater computational power, and the abundance of training data, deepfakes have now reached a level of realism that often makes them indistinguishable from authentic content. These deep learning systems are now remarkably proficient at replicating human features, expressions, and voices with unnerving accuracy.
In response to the growing impact of deepfake technologies, the Lawyers Hub hosted a session titled "A Survey on Deepfake Technologies" at the Africa Law Tech Festival 2024. This article will explore the key discussions, insights, and recommendations shared during the session.
Session Overview
Deepfakes: What They Are and How They Came About
Deepfakes are synthetic media created using artificial intelligence, specifically through deep learning techniques, to produce highly realistic but fake content. The term itself is a combination of "deep learning," a subset of AI, and "fake." Deepfakes typically involve manipulating images, videos, or audio to make it appear as though someone is doing or saying something they never did. For instance, in videos, deepfakes can swap faces, manipulate expressions, or even generate entire fake scenarios.
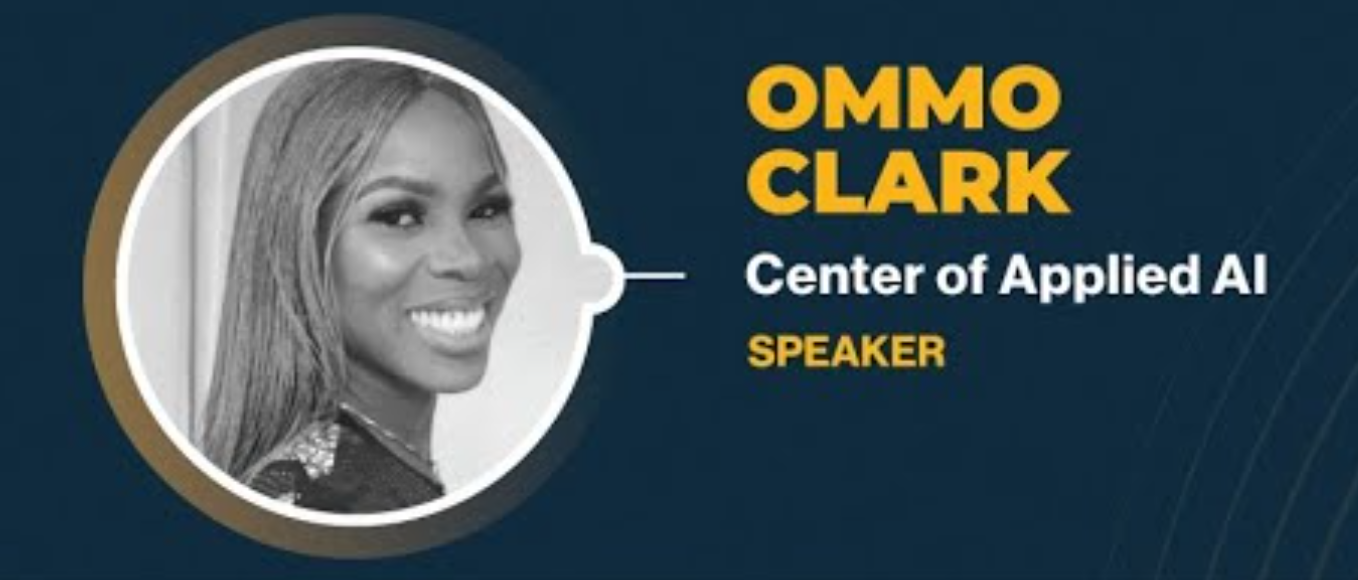
Origins and Evolution of Deepfakes
The roots of deepfake technology date back to the 1990s when CGI (computer-generated imagery) was being explored to create realistic human likenesses. However, it wasn’t until the 2010s that deepfakes became much more advanced, thanks to the availability of large datasets, improved machine learning algorithms, and enhanced computing power. A significant turning point came in 2014 when Ian Goodfellow and his team introduced the concept of Generative Adversarial Networks (GANs), a machine learning model that enabled the creation of more sophisticated deepfake images, videos, and audio.
In 2017, the term "deepfake" was coined by a Reddit user who popularized a subreddit dedicated to creating and sharing deepfake videos, specifically those that used AI techniques to swap celebrity faces into adult content. Although the forum was later banned, the term stuck and became associated with the rise of AI-generated fake media.
By 2018, concerns over deepfakes were growing rapidly due to their increasingly realistic nature and potential for misuse. This led major tech companies to roll out policies aimed at moderating and detecting deepfakes on their platforms. Reality Defender, initially a nonprofit, also emerged during this time as a key player in deepfake detection.
The following year, in 2019, saw further developments. Countries like the United States began exploring legislative measures to regulate deepfakes. At the same time, deepfake technology spread globally, with AI-generated ads and websites like "This Person Does Not Exist," which created entirely synthetic but realistic human faces, further fueling concerns about the misuse of the technology.
By 2022, deepfake audio also became more advanced, allowing for highly realistic voice impersonations. During the Russian-Ukrainian conflict, deepfakes were even used as tools for spreading misinformation. As of 2024, deepfakes have become so sophisticated that real-time deepfake generation is possible, where images of individuals can be manipulated almost instantly, demonstrating how far the technology has progressed.
Widespread Use Across Industries
Today, deepfakes are prevalent across a wide range of industries:
Entertainment: Filmmakers and content creators use deepfakes to de-age actors or create realistic digital doubles, allowing for seamless visual effects.
Medicine: In healthcare, deepfakes can simulate complex medical procedures for training purposes, offering life-like, hands-on learning experiences for medical students.
Education and Training: In both fields, deepfakes help simulate real-world scenarios that allow learners to practice complex tasks in a safe, controlled environment.
The Rising Demand for Deepfakes and Market Growth
The deepfake market is experiencing rapid growth. According to the speaker, in 2024, it was valued at approximately $7 billion, with projections suggesting it could soar to $38.5 billion by 2030. This reflects increasing demand for both legitimate applications, such as training and entertainment, as well as malicious activities like misinformation and fraud.
According to the Global Deepfake AI Market Size, Share, and Growth Analysis by Offering (Deepfake Detection & Authentication, Deepfake Generation, Services), Technology (GAN, NLP, Autoencoders, Diffusion Models, Transformers), Vertical and Region - Industry Forecast to 2030 report, the deepfake AI market alone was estimated at $562.8 million in 2023, expected to reach $764.8 million by 2024, and is forecasted to grow at an impressive compound annual growth rate (CAGR) of 41.5% through 2030, reaching $6.14 billion. This expansion is driven by advancements in generative AI, improved deepfake detection, and the rising demand for realistic, customizable digital content across various industries.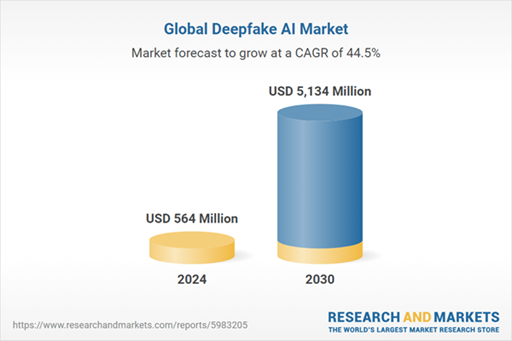
The increasing commercial potential of deepfakes across industries also highlights the urgent need to manage their risks. From fake news to identity theft, the misuse of deepfake technology poses significant threats to individuals, corporations, and governments.
Risks of Deepfakes
Deepfake technology has become a significant risk, particularly in financial fraud, misinformation, and privacy violations. A recent case in Hong Kong highlights the threat, where a finance worker was deceived into transferring $39 million after being tricked by deepfake impostors posing as their CFO and colleagues. This incident underscores how scammers are leveraging deepfakes to mimic voices and appearances, making financial fraud harder to detect.
Beyond financial crime, deepfakes are used to damage reputations by spreading lies and fabricated content. Alarmingly, non-consensual pornography, accounting for up to 96% of all deepfakes online, targets mostly women and celebrities. Deepfakes have also been weaponized in social engineering scams. In one instance, the CEO of a U.K. energy firm was fooled by an audio deepfake mimicking the chief executive of their parent company, resulting in the transfer of €220,000 to a fraudulent account.
Political manipulation is another serious concern. Deepfake videos of world leaders have the potential to spread disinformation, disrupt elections, and fuel conspiracy theories. One infamous example is a deepfake video of Facebook founder Mark Zuckerberg claiming to control billions of people’s data through a fictional entity from James Bond stories.
The technology also facilitates identity theft. Attackers can use deepfakes to falsify documents, imitate a victim’s voice, and create fake identities, enabling them to open accounts, make purchases, or commit fraud. As AI technology advances, deepfakes pose an ever-increasing threat to privacy, security, and trust in digital interactions.
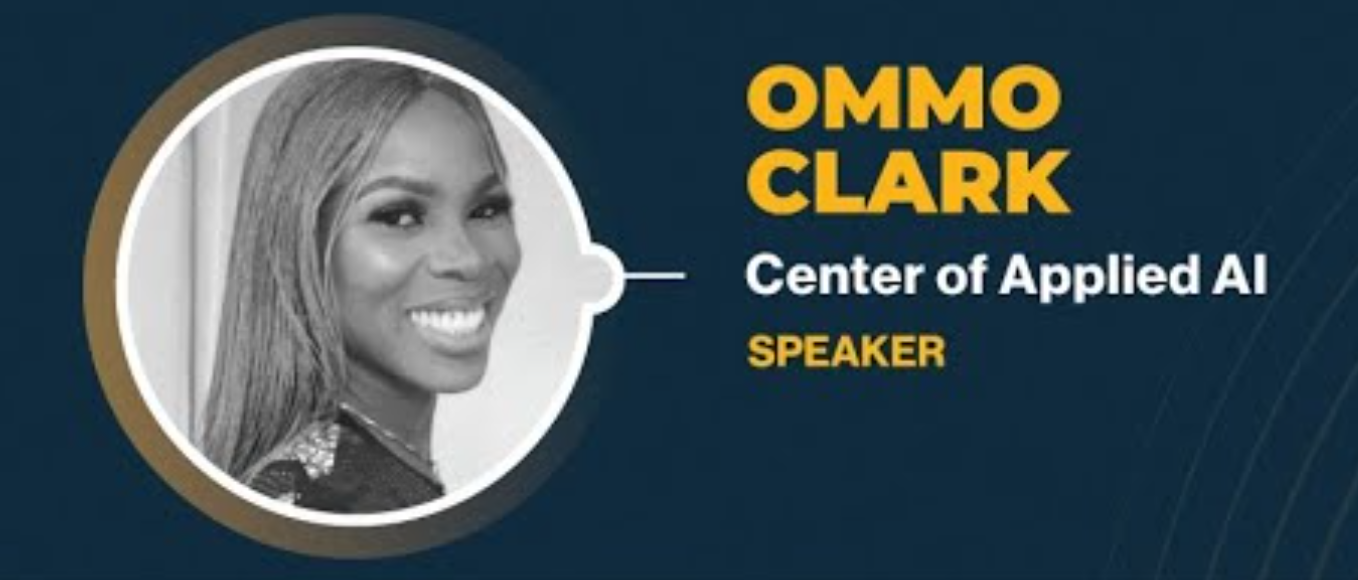
Survey Highlights Gaps in Deepfake Detection Tools: A Growing Need for Improvement and Standardization
With the rise of deepfake technology the need for robust detection tools has never been greater. These tools aim to identify deepfakes, helping prevent the spread of misinformation, fraud, and other malicious activities. However, recent research into the effectiveness of deepfake detection solutions, a survey highlighted by the speaker, has revealed significant gaps in the current landscape, suggesting that more work is needed to keep up with the fast-evolving technology behind deepfakes.
Purpose of the Survey
According to the speaker, the objective of the survey was clear: to evaluate the effectiveness of existing deepfake detection tools, understand the state of the market, and pinpoint areas for improvement. The survey selected 35 tools based on mentions in media and academic research. From this group, they tested a sample to verify the tools' performance and self-reported accuracy rates.
Key Findings
While the research team initially identified 35 tools, only 30 were specifically focused on detecting deepfakes. Others addressed related areas, such as narrative tracking and AI-generated content detection, which can also help monitor trends like misinformation or academic integrity issues.
A core finding was the variation in performance across the tools. Many struggled to accurately detect more sophisticated deepfakes, especially when compared to their self-reported accuracy rates. Some tools claimed up to 99% accuracy, but third-party verification was lacking, and no industry-standard benchmarks currently exist to assess and compare their performance.
Challenges in the Current Landscape
The maturity of the deepfake detection market was another key issue. Many of the companies behind these tools were relatively young, with some less than a year old. This often meant that their products were still in early development stages, lacking the comprehensive capabilities needed to tackle the growing threat of sophisticated deepfakes.
Accessibility also varied widely. Roughly half of the tools offered free or open-source versions, which could be a positive for those with limited budgets. However, for others, access came at a cost, with subscription fees potentially placing reliable detection solutions out of reach for smaller organizations or individuals.
Media-Specific Detection Issues
Most tools focused on detecting video-based deepfakes, as these are currently the most prevalent form of deepfake content. However, audio deepfakes, which are widely regarded as more difficult to detect, were largely neglected. Only a few companies offered solutions targeting audio-based deepfakes. Similarly, text-based detection tools, particularly in areas such as AI-generated content or academic integrity, were rare.
An additional challenge was the lack of multimodal detection—tools that could detect deepfakes across different media types, such as video, images, audio, and text. Only a handful of the tools surveyed offered this capability. This is problematic for users who need to monitor for deepfakes across various types of media, as they may need to rely on multiple tools, which increases complexity and cost.
Accuracy and Inconsistency
Across the tools tested, accuracy levels were inconsistent, and none of the solutions matched their self-reported accuracy rates. While two tools came close, most fell short. Performance varied depending on the type of media, with some tools performing better on specific types (e.g., video or image) but struggling with others. This inconsistency was often tied to the maturity of the tools, with more established ones performing better than those still in early development stages.
Areas for Improvement
According to the speaker, the survey highlighted several key areas where deepfake detection tools need to improve:
Cross-media detection: Tools need to be capable of detecting deepfakes across multiple types of media, including video, images, audio, and text. Multimodal detection is essential to address the diverse range of deepfake content.
Real-time scalability: As deepfakes become more sophisticated, detection tools need to keep pace. Real-time detection at scale is crucial for combating the rapid spread of misinformation and other malicious content.
Continual updates: The deepfake landscape evolves quickly, with creators often staying ahead of detectors. Detection tools must be continuously updated to handle new types of deepfakes as they emerge.
Ethical considerations and bias: Some tools struggled to detect deepfakes involving accents or diverse linguistic patterns, revealing potential bias in the training data. This highlights the need for more inclusive, global datasets to ensure that detection tools are effective across different languages and cultures.
Future work needed
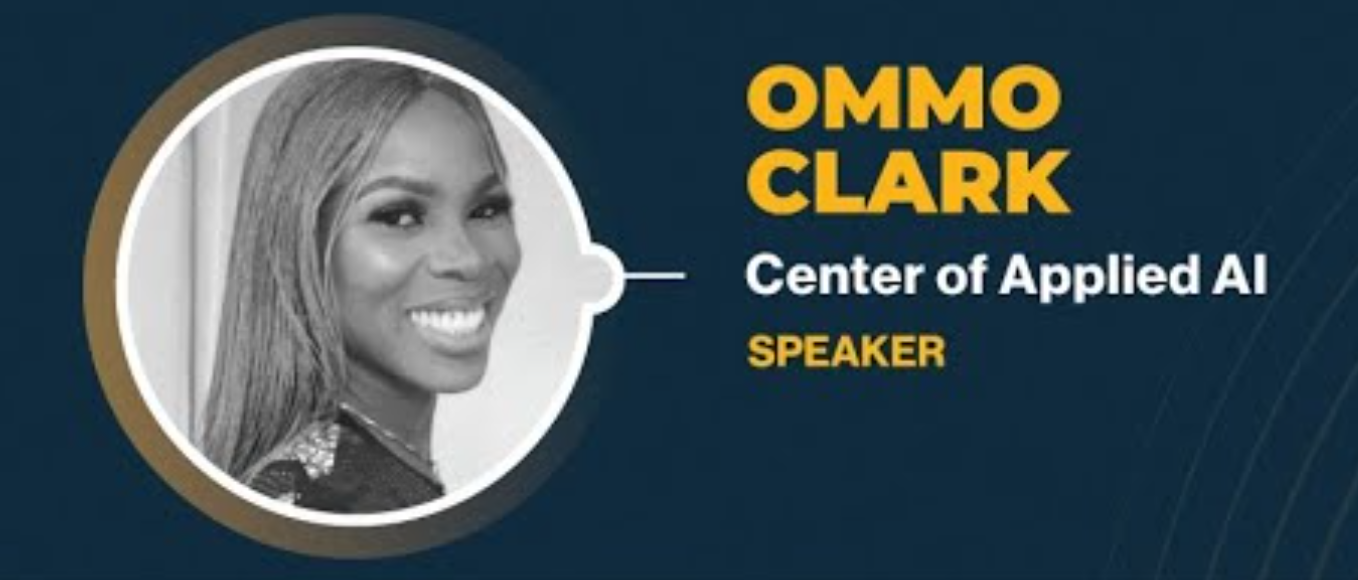
- Need for standardized benchmarks
- Increased Investment in Research and Development
- Reliable test datasets are needed
- Need for Deepfake Literacy
- Tools need to be further tested when they are more mature.
Conclusion: The Road Ahead
The deepfake detection market is still in its early stages, with many tools showing promise but struggling to deliver consistent accuracy. The fast pace of advancements in deepfake creation technology means that detection tools are constantly playing catch-up, making it crucial for developers to continue improving their solutions.
Future progress will require standardization. Industry benchmarks are needed to evaluate deepfake detection tools accurately and consistently. Moreover, investment in research and development is critical to ensure that detection tools can keep pace with increasingly sophisticated deepfakes.
In addition, deepfake literacy needs to be part of the conversation. As deepfakes become harder to detect, educating the public about the potential dangers and how to critically assess digital content will be essential. Just as digital literacy became crucial in navigating the internet age, deepfake literacy will help individuals discern between real and manipulated media in the era of AI.
Ultimately, deepfakes are dangerous, but with continued research and investment, detection tools can improve. By addressing the challenges identified in this survey, the field can develop reliable solutions capable of effectively combating the risks posed by manipulated data.